Data Quality is vital for organisations that produce and consume large amounts of data, as outlined in our previous blog. Enterprise-wide data quality management requires a dedicated data quality tool to make sure data is fit for purpose. This blog outlines why you need a data quality tool, what capabilities to look for in a data quality tool and highlights three different data quality tools from different vendors.
Assessing quality in a digital world
Just like manufacturing businesses, which carefully inspect their products to ensure they meet quality standards before reaching the consumer, organisations that aim to be data-driven must carefully assess the quality of their data. Just as a flaw in a product can lead to significant repercussions for a company, inaccuracies or inconsistencies in data can result in flawed analysis, misguided decisions, and ultimately, damaged reputations.
However, unlike tangible products whose defects are often visible or easily detectable, the challenges of identifying data issues are far more complex. In order to guarantee that data is suitable for its intended purpose and aligns with the needs of data consumers, it is essential to assess and monitor its quality. Without systematic measurement and evaluation, hidden inaccuracies, duplications, inconsistencies, and gaps within datasets can go unnoticed, potentially undermining the entire foundation of decision-making processes.
Monitoring data quality entails employing appropriate tools to oversee data quality continuously. Data Quality tools like Collibra Data Quality, Informatica, or Soda serve as indispensable assets, providing organisations with the means to measure, monitor, and improve data quality systematically.
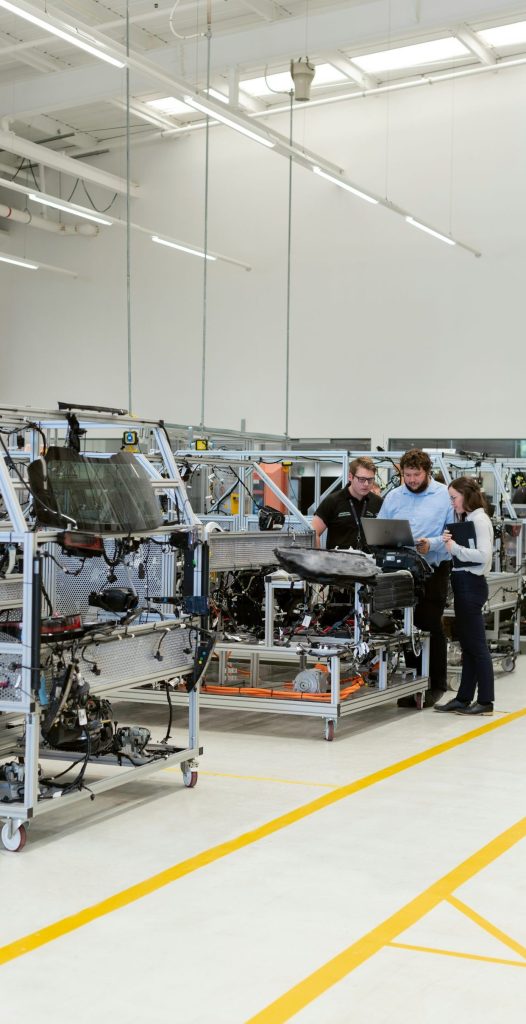
Three common capabilities
The most common capabilities provided by data quality tools are profiling, rule enforcement, and dashboarding.
Data Quality Profiling
Profiling serves as a cornerstone in the data quality process by providing essential statistics on various data attributes. Profiling provides insights in basic data attributes such as data types and percentages of null values or empty fields. By finding mean, maximum, and minimum values as well as quartiles, the distribution of numeric values is described. Profiling is used to establish baseline metrics and to pinpoint anomalies.
Data Quality Rules
Business rules guide organisational operations and can be transformed into data quality rules for assessing the quality of data. Coupled with data quality dimensions, these rules provide a structured framework to evaluate aspects like accuracy, completeness, consistency, and timeliness of data. This alignment ensures data remains reliable and actionable, supporting informed decision-making. Data Quality tools allow users to check the quality of their data based on their self-defined rules.
Data Quality Dashboarding
When measuring data quality, it is helpful if a data quality tool is able to present findings on a dashboard. A data quality dashboard should offer a concise and comprehensive snapshot of the quality of the data that was measured. It should also identify faulty records so data stewards can analyse defects and find a starting point for data remediation. Most data quality tools come with an out-of-the-box dashboard, and many allow their users to also build their own dashboards.
Data Quality tool spotlight
A variety of Data Quality tools can be found on the market. In this section we discuss three Data Quality tools from well-known vendors: Collibra, Informatica, and Soda. We have highlighted three unique strengths of each tool!
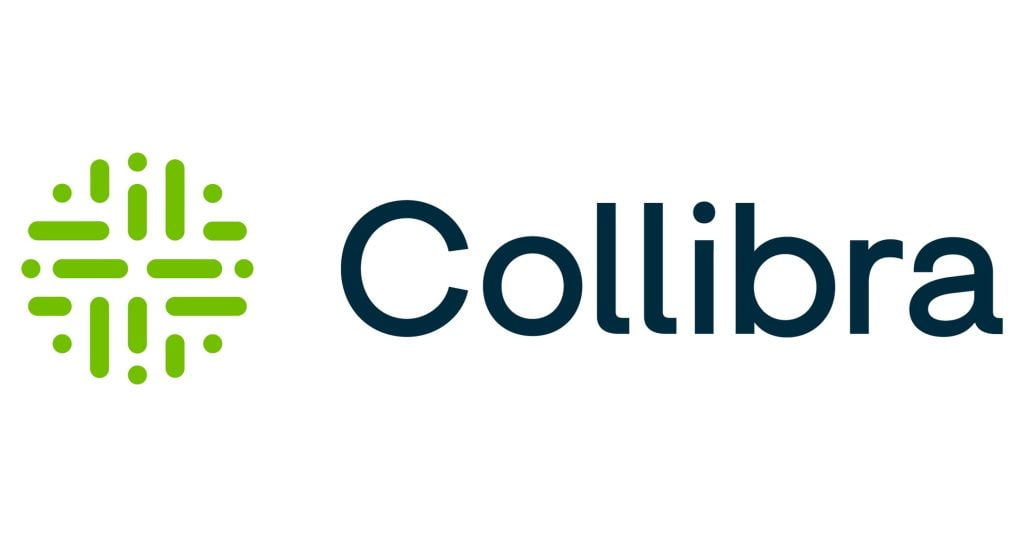
Collibra Data Quality & Observability is Collibra’s dedicated Data Quality tool. Its offer includes profiling, custom data quality rules, data pipeline monitoring, and data quality dashboarding, making it a solid data quality tool. Collibra DQ can connect to a big range of databases and file storage systems, which makes it deployable in most data landscapes. Collibra DQ stands out in a few areas:
- Machine learning-based, automatic rules. Collibra DQ has the capability to monitor data over time and learn about the behavior of datasets. Based on this behavior, it automatically generates a large amount of data quality rules. This saves a lot of time spent manually writing data quality rules. Collibra DQ automatically detects and reports records that are breaking these automatic rules.
- SQL-based custom rules. In Collibra DQ, data quality rules are written in SQL, which is a widely adopted language for querying databases. This makes writing Data Quality rules accessible for anyone who knows the basics of SQL. Collibra DQ has an AI capability that helps users with rule-writing, although this feature currently is still in beta.
- Integration with Collibra Data Intelligence Platform. Collibra DQ integrates nicely with Collibra’s main product, the Data Intelligence Platform. Data quality scores measured in Collibra DQ can be mapped to the corresponding assets in Collibra DIP’s data catalog, making the quality of data visible for browsing users. Collibra is moving towards further developing this integration in 2024. For organisations that already use Collibra DIP and want to adopt a data quality tool, Collibra DQ is the top choice.
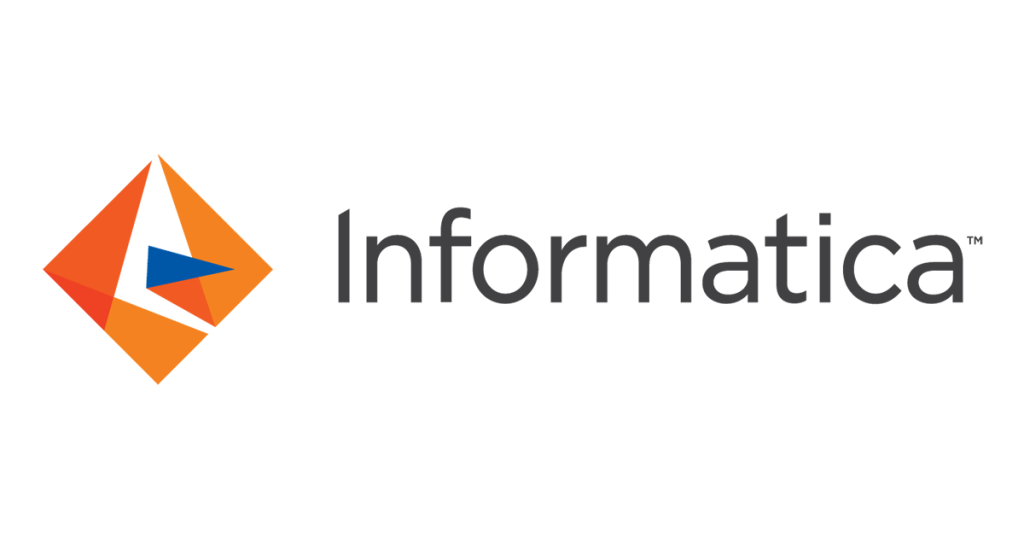
Informatica Cloud Data Quality provides organisations with the tools needed to effectively manage and enhance their data quality in real-time, empowering them to make informed decisions and derive valuable insights. Here are three notable capabilities that set it apart:
- Advanced data profiling and assessment: The platform offers robust data profiling tools, allowing organisations to gain deep insights into the quality of their data. By analysing data from multiple sources, it helps identify inconsistencies, inaccuracies, and anomalies. Through intuitive dashboards and visualisation features, users can comprehensively assess the health of their data, enabling them to prioritise areas for improvement effectively.
- Efficient data standardisation and cleansing: Informatica Cloud Data Quality excels in automatically standardising and cleansing data using its extensive library of pre-built rules and algorithms. Whether it’s correcting errors, removing duplicates, or ensuring compliance with data governance policies, the platform streamlines the process of enhancing data quality. It offers comprehensive support for parsing unstructured data, validating addresses, and enforcing standardised formats, ensuring that data remains accurate and consistent across the board.
- Real-time monitoring and remediation: One of the key strengths of Informatica Cloud Data Quality is its ability to monitor data quality in real-time and take proactive remediation actions. Through continuous monitoring and alerts, organisations can identify data quality issues as they arise, minimising the impact on business operations. The platform enables users to set up thresholds and automate remediation workflows, ensuring that data quality standards are upheld consistently over time.
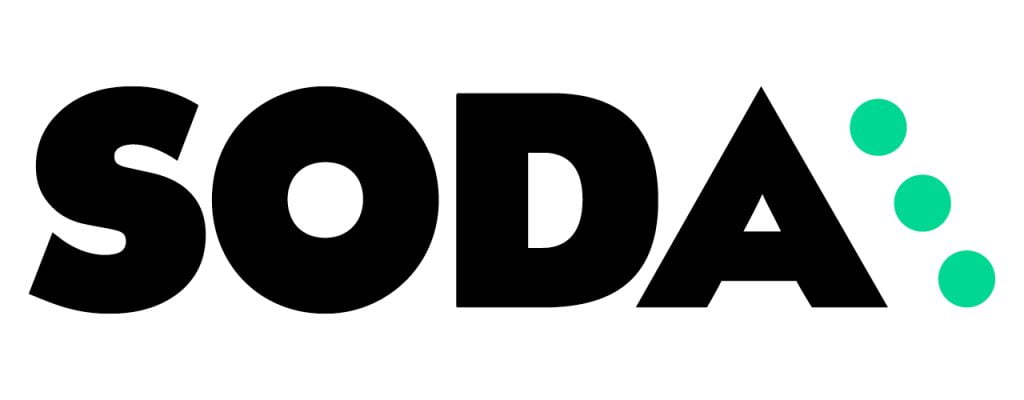
- Wide range of integrations. Soda has a wide array of integrations with not only data warehouses, but also to data pipeline orchestration tools such as Airflow and visualisation tools such as Tableau. This flexibility makes Soda easy to integrate with the existing data stacks of data-driven organisations.
- SodaGPT uses AI to help non-technical users with rule-writing. When it comes to leveraging AI to help non-technical users write data quality rules, Soda is definitely a frontrunner. SodaGPT takes natural language as input and provides data quality checks in Soda Checks Language (SodaCL), Soda’s own language for data quality checks. This makes writing data quality rules accessible to a broader audience while accelerating the workflow of seasoned data stewards and engineers.
- Data quality collaboration. Soda offers in-tool discussion boards to streamline collaboration between data engineers and data owners. Furthermore, its integrations also include collaboration tools like Slack, Microsoft Teams, and Jira. For example, a specific data quality issue in Soda can be linked to an issue in Jira allowing organisations to utilise their existing incident management workflows in Jira for data quality management.